Quantitative Finance - Definition and History
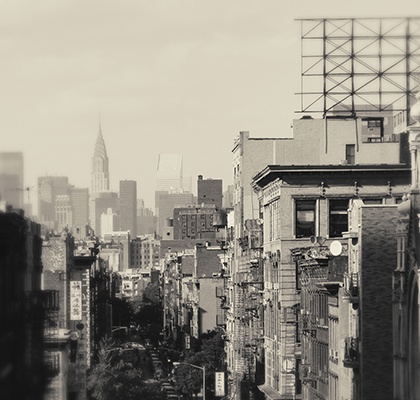
What is Quantitative Finance?
Quantitative finance is a branch of investment management that employs mathematical and statistical methods to analyze investment opportunities across a range of asset classes. Practitioners in quantitative finance (“quants”) work in equities, fixed income and structured products, commodities, foreign exchange, and all varieties of derivatives. Areas of sub-specialization include derivatives pricing, trading and hedging, portfolio analysis and optimization, risk management, and regulatory compliance. In addition, quants are entering the world of artificial intelligence and machine learning these days, where the demand for data scientists in the financial industry is growing dramatically.
The History of Quant Finance
Quantitative finance arose in the mid to late 20th century as the financial markets evolved and became larger and more complex. However, some of the key models and theories appeared even earlier. Explore an outline of some of the key quant finance milestones and practitioners below.
The 1800s
The early 1800s
In 1827, one of the main concepts in quant finance originated in the field of biology when Robert Brown, a Scottish botanist, observed the movement of particles trapped inside grains of pollen on a pool of water. He noted the seemingly random patterns these particles moved in. This random movement become known as “Brownian motion,” a cornerstone of quant finance, along with the notions of drift and diffusion.
The late 1800s
In the 1860s, Jules Augustin Frédéric Regnault used the concept of the “random walk” to explore the modern theory of stock price changes in his book, Calcul des Chances et Philosophie de la Bourse. Regnault, a French stock broker’s assistant, was one of the earliest writers to create a ‘stock exchange science’ based on statistical and probabilistic analysis.
The 1900s
The early 1900s
A major milestone for the world of derivatives was set in 1900 by Louis Bachelier, a French mathematician, with the publication of his PhD thesis, ‘The Theory of Speculation’, in which he modeled the stochastic process now called Brownian motion and used it to evaluate stock options. Bachelier is credited with being the first person to do this and his Bachelier model has been pioneering in the development of other widely used models, including the Black-Scholes model.
English mathematician and biostatistician, Karl Pearson, introduced the term “random walk” in 1905. Pearson is largely credited with establishing the discipline of mathematical statistics and themes from his book, ‘The Grammar of Science’, was later used in the theories of Albert Einstein and other scientists.
By the mid-1900s, economists were laying the foundation for a more developed theory of financial markets, with the work of, Friedrich Hayek, on efficient markets in the 1940s, and the emergence of Modern Portfolio Theory following the publication of Harry Markowitz’s thesis “Portfolio Selection” in 1952. Modern Portfolio Theory is now widely used by institutional investors and financial advisors for asset allocation, risk control, and attribution analysis. In 1990, Markowitz went on to win the Nobel Prize in Economic Sciences for his work on portfolio theory.
The late 1900s
In the early 1970s, American economist, Eugene Fama, built on this foundation and advanced the field with his efficient market hypothesis (EMH) in the early 1970s. Fama is often referred to as the ‘Father of Finance’ as his work developed much of the groundwork for financial economics today. Since Fama’s work, the development of a diverse range of quantitative models has been a natural progression for those seeking to analyze stocks, assess the efficient risk-reward frontier, and engage in portfolio optimization.
Other figures central to the evolution of quant finance in the late 1900s include Edward Thorp, a mathematician who came to finance through his work on probability and statistics as deployed on the blackjack tables of Las Vegas. Thorp’s book, ‘Beat the Dealer’, published in 1966 explored his blackjack game theory and is often considered the original guide to card counting.
Another notable quant of this period was Emanuel Derman, one of the first physicists on Wall Street, who developed a number of models that remain in use today – including the Black-Derman-Toy model (BDT). With the rise of option pricing and quant trading in the 1970s and 1980s, following the work of Fischer Black, Myron Scholes, and Robert Merton on the eponymous Black Scholes (Merton) equation in 1973, quant methods and advanced computing became ubiquitous in the derivatives market.
Quant Finance Today
Today, quant finance approaches are applied in all markets, from equities and bonds to structured products, derivatives, commodities, and even approaches to venture capital and private equity investment. Models have proliferated, with critiques, improvements, implementations, and performance analysis in papers and journals, beyond the results coming from the market itself.
In addition to the advancement in models and mathematical methods for quant analysis, technology has played a very important role in the evolution of quant finance. In the early 1990s, electronic trading platforms were introduced and supported the global expansion of the stock, bond, and credit markets through the 2000s. Further, the spectacular growth in the volume, velocity, and variety of data has driven intense activity in machine learning from the 2010s to the present.
In addition to these trends, hybrid forms of investment analysis are emerging. While quantitative analysis is distinct from fundamental analysis, with its focus on the valuation of individual stocks, in the field of “quantamental” analysis, techniques from fundamental research are combined with quantitative methods to form judgments about investment opportunities.
Quant methods are also being applied to ESG investing, particularly around score methodologies, and machine learning is being employed in alternative data analysis, speech and natural language processing (for earnings calls and Twitter sentiment, for example), and signal processing from tick data – getting down inside the market microstructure itself.
Each of these research areas requires in-depth knowledge of quant models and methods, strong computer programming skills, and a good understanding of the financial markets. So, what should an aspiring quant do?
Quantitative Finance and the CQF
Quant finance is a demanding field with excellent opportunities for curious, highly motivated people. Roles in quant finance offer good salaries, opportunities for growth, and considerable job satisfaction for people who are truly interested in the real world of the financial markets. Niches like cryptocurrencies and ESG investing are also drawing on quant talent and the development of new tools, products, and services will provide further opportunities for quants in the future.
Such dramatic changes in the financial markets underscore the importance of continued professional development throughout your career. The Certificate in Quantitative Finance (CQF) program is a globally recognized, master’s-level professional qualification. Delivered online by world-renowned quant practitioners, the program teaches the cutting-edge practical quant finance and machine learning skills used in today’s financial markets. Upon completion of the program, all CQF alumni also have permanent access to the ever-expanding Lifelong Learning library which includes talks and masterclasses from industry experts and all of the updated CQF program content.
As the markets continue to evolve, education in quantitative finance is a wise investment at any stage of your career to help you attain your professional goals in the years to come.