The Importance of Machine Learning for Risk Management
April 2021
How does machine learning fit into financial services?
In recent years, machine learning (ML) has become an important component in a range of financial services contexts, from asset management, algorithmic trading, and robo-advising to credit evaluation, loan decisions, and fraud detection. Machine learning is well-suited for analyzing transactions, searching for alpha signals, and assessing patterns of behavior drawing on the tremendous volumes of data generated in the investment and banking industries. As the tools and techniques have gained traction, many firms are incorporating machine learning capabilities into their business, trading, and risk management processes.
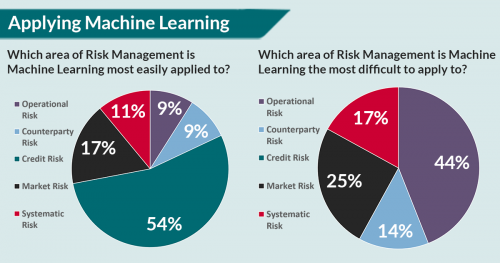
Risk management and ML: detection, prevention, and prediction
One prominent use case for ML in risk management is fraud detection and prevention. Credit card fraud, for example, is a significant concern for financial services firms. Machine learning can help detect and flag fraudulent transactions and anomalies, first by learning and retaining a profile of typical consumer behavior and then comparing a current transaction in-process against the set of known data points, including geographical locations, IP addresses, account history, and other customer information. If an intended purchase or withdrawal seems suspicious, the system can decline to complete it, notify the institution and the customer, and wait for validation.
Machine learning is also being applied to credit risk modeling, using traditional credit factors, including debt-to-income ratios, banking information, and credit card history. The holistic view – taking a deeper look at patterns of consumer behavior – enhances the predictive power of the models. Extending from the individual case to the corporate case, machine learning is useful in compliance through parameter optimization in regulatory models. As in the case of credit card fraud, machine learning is also being applied to highlight potential money laundering activities, in support of human evaluation and escalation efforts when questionable behavior has been discerned.
CQF Institute poll highlights the importance of ML for risk management
Concerning the adoption of ML across the financial services industry, in a poll run by the CQF Institute, 42% of all respondents expressed a strong view that machine learning for risk management is an extremely important topic; 32% said it was important and 19% said it was critical.
In terms of the type of ML favored at this time 44% indicated a preference for supervised learning as the leading technique at the moment, with unsupervised learning and reinforcement learning tied at 28% each.
Credit risk was viewed as the easiest area for ML applications (54%), followed by market risk (17%) and systematic risk (11%), although the latter two are also seen as being the most difficult areas to handle, along with operational risk and counterparty risk, which were tied at 9% each.
Finally, 44% of all respondents said their firms were thinking about applying ML in specific areas, although only 28% are actively doing so. Looking across all areas of risk management, 6% said their firms were applying ML broadly, and another 6% were thinking about proceeding with such sweeping application. Time will tell how the incorporation of ML unfolds.
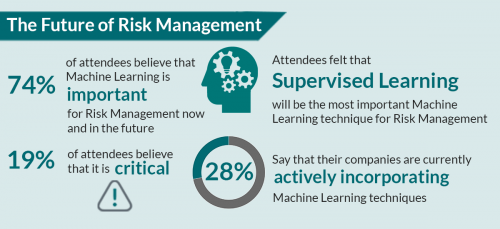
The Certificate in Quantitative Finance and ML: An evolving curriculum
Due to the complexity and interdisciplinary nature of machine learning, quant skills are in very strong demand in financial firms and technology firms that sell to financial market participants. Candidates who can cover all the bases: statistics, math, finance, and programming have a distinct advantage in these job markets around the world. Furthermore, university education in these areas typically lacks practical grounding and critiques; even the best courses may not match up with current industry knowledge and practice. So, how can the interested and ambitious professional proceed on a path through machine learning for finance?
The Certificate in Quantitative Finance (CQF) program, delivered part-time and online, is taught by seasoned industry experts and equips delegates with core skills through an up-to-date and evolving curriculum designed to meet the challenges and opportunities in quant finance today. Beginning with optional primers which serve as a foundation in mathematics, Python programming, and finance, the CQF proceeds module by module through portfolio theory, principles of risk and return, and core asset classes including equities, currencies, fixed income, and credit. In each segment, classic quant models and methods are presented, explored, and critiqued, with ample time devoted to practical application through problem sets, further reading recommendations, and exams.
For each of these areas of quant finance, data science and machine learning are driving new insights in historical analysis, back testing, simulation, and prediction. These topics are all covered rigorously in the CQF program, with an emphasis on industry practice. In the first of two modules on machine learning, the core topics include supervised learning, regression methods, k-nearest neighbors, support vector machines, and ensemble methods. The second module addresses unsupervised learning, deep learning, and neural networks, with a look at natural language processing and reinforcement learning. The course work encompasses both the theoretical framework behind the programming and real-world case studies that focus on how ML techniques are applied to problems in finance.
For those seeking a broad and deep background in quant finance – from models and methods to machine learning in the current financial and economic environment, the CQF program offers a timely, flexible, and high-quality professional education, with a strong focus on asset management, risk management, data science, and machine learning. For those who embark on this journey, the CQF will provide a foundation for pursuing the most interesting and challenging opportunities in the quant finance industry today and into the future.
To find out how you could transform your career with the CQF program, please download a brochure or join the next online information session to hear more and ask questions to the CQF Program Director.