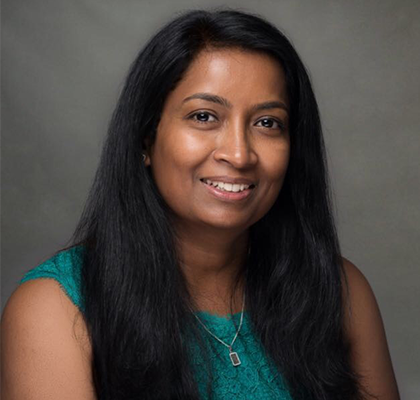
Chelvi Paramanathan, Global Head of Pricing and Analytics, Agency Securities Finance and Collateral Services, J.P. Morgan Chase & Co.
Chelvi earned the CQF in 2018. Chelvi started her career as an engineer before transitioning into finance. We spoke to Chelvi about making that transition and her advice for people looking for their next quant role.
How did you decide to pursue your current career path?
My childhood dream was to become a software engineer, so I studied Electronics and Electrical Engineering as an undergrad, and then completed a post graduate degree in Artificial Intelligence. For work, I started as an engineer in the scientific research industry in Singapore while pursuing my master’s in Knowledge Engineering/AI. The research lab was funded by the Singapore government, and I was fortunate to work on many high-profile projects there. This gave me fundamental and practical programming skills and application of AI, but I have always been passionate about numbers too and I looked for an opportunity to do both. I found this in finance and took a job where I was attached to a long-dated volatility trading desk. The team was working with exotic options, and I built option pricing and risk management systems. Very quickly I developed a deep interest in learning more about application of math in finance, so I did master’s level credit programs in finance, focusing on risk management, capital market portfolio theory, and financial mathematics. This helped me to secure a front office role and I moved into quantitative research at Lehman Brothers, where I was attached to a desk and built models for business efficiency and price discovery.
That is clearly a lot of education! How did you discover the Certificate in Quantitative Finance (CQF) and how did it fit into your plan?
At that point in time, I was contemplating a CPA or a CFA, but neither of them was deeply focused on the quant side of things. Then I came across the CQF through the program’s website, and it was precisely what I was looking for. I felt that I would not only get a certification in quantitative finance, but I would also learn how to work with quantitative models and apply AI techniques. The CQF has had a significant impact on my career because in working with all kinds of risk: counterparty credit risk, market risk, portfolio construction and optimization and building various financial models, it gave me the background and confidence to come up with solutions that have quantitative proofs and help with decision making or solve client’s problems with quantitative evidence.
What kind of work is involved in your current role on a day-to-day basis?
I have built a global team that evaluates client portfolios in the securities financing business. I'm also responsible for data analytics and innovation initiatives for the business, so I have a group of data scientists and quantitative researchers generating new ideas and tools to drive business efficiency. I work closely with our functional partners in technology, product development, and quantitative research to build end-to-end solutions. Finally, I'm responsible for managing the financial resource usage of our business, which is the capital footprint for the business under Basel III guidelines and any changes in the Basel III endgame impacting the business.
If you look out over the markets, what are some of the biggest challenges over the next few years?
Working with data. When I started in finance, we only had our own proprietary data, and we didn't have extremely powerful desktops or tools at that time. Running a Monte Carlo simulation on a long dated exotic option trade requires multiple Sun Sparc machines and overnight batch process. Now the sky is the limit and sophisticated IDEs and cloud computing made compute intensive calculations and big data mining more time efficient and infrastructure efficient. Mining large sets of complex data with traditional low level programming language takes signification amount of time to cleanse data to remove noise to extract curated data to be used in the analytics to find meaningful signals and that's a challenge, but with the tools, ample compute power and machine learning techniques available today, you have sufficient resources to deal with the situation. It's also becoming much more complex and competitive given the availability of large sets of data from various sources and in various formats, you must develop your own insights and algorithms to get the meaning out of the data. Endless learning and experience in programming starting from low level programming languages like C, C++, and java to using these rapid development tools like Python and R, gives a competitive advantage due to the wealth of knowledge that you have gained over time.
What would you advise for people who are in the job market these days?
Staying current in your field is essential. I stay up to date with the markets and continue learning in various areas through online certification programs, and I recommend being committed to expanding your domain expertise regularly. Based on my experience, I feel that we drive our own careers, so we must have passion and dream or envision the path to achieve that goal. And stay focused. In five years’ time, where do you want to be and how will you get there? Some other observations are that communication skills (soft skills) are very valuable and working effectively on a team is as well. You're still going to face lots of obstacles along the way but keep working towards your goal and spend time on the business skills as well as the more technical capabilities.
That is great advice – motivation and drive are important factors in work and in life! Are there any specific skill sets along the horizon that people should consider?
Machine learning, in its various forms, including deep learning techniques is important. However, AI is not always fully applicable to every domain, so it’s good to be able to critique the methods and the potential shortcomings. That said, programming ability in Python or R is essential along with familiarity of big data platforms like Spark, and people should be aware of the evolution of these tools. Looking back, I started with C, then came Java, which is a wrapper on top of C. Then JavaScript on top of Java and now we have Python. At the end of the day, the fundamental coding concept is more or less the same but made it easy with readily available open-source toolkits and libraries but having core programming skills and the ability to tell a story with data will make a huge difference. Just have passion for your work and stay focused: the opportunities will be there.
Find out more about the CQF program
To discover how the CQF program could help you go further in your career, download a brochure or register to join an information session.