In Conversation with CQF Alumni: Charlie Flanagan
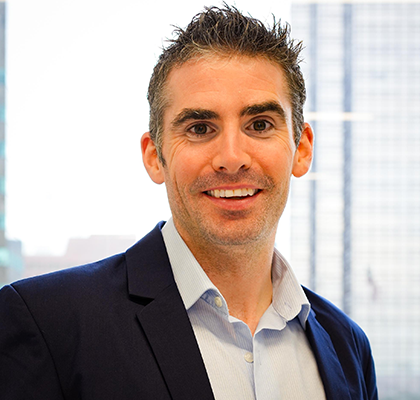
Charlie Flanagan, Head of Applied AI, Balyasny Asset Management
Charlie earned the CQF in 2014. He started as an Equity Derivatives Dealer before becoming Head of Applied AI for Balyasny Asset Management. We spoke to Charlie about the necessity of learning programming languages like Python.
Let’s start with your education and early career. Tell us about how you became interested in quantitative finance.
I started my career wanting to become an Equity Analyst. I grew up in Ireland, did an accounting degree at Galway-Mayo Institute of Technology, and then moved to London. I started working for ICAP, a broker-dealer, where I became interested in derivatives. I got a placement on the equity options desk, which was my first exposure to the world of quantitative finance. I worked on that desk for about two years and during that period, I read many of the “bibles” of options theory on pricing models and the heuristics around options and trading. Then, I had an opportunity to join the hedge fund, QuantRes, in the Bahamas. They focused on deep quantitative research, and I had an opportunity to learn from some incredibly talented quants and engineers. I was there for about seven years and completed the CFA program during this time. However, I felt I needed get into more depth on quantitative methods, so I enrolled in the CQF.
You had been in the business world for quite a few years at that point. When you were deciding on which professional designations to pursue, what were your main considerations?
There were several considerations. The first was an interest in gaining rigorous training in quantitative methods. During my undergraduate degree, I took some operations research classes, but I didn't have formal education in a quantitative discipline. So, after my experience in London and the Bahamas, I wanted to expand my understanding beyond equity options pricing, which had been my professional focus until then. The CQF allowed me to explore interest rates and credit, for example.
A second motivating factor was the opportunity to meet other quants in the field. I was still in the Bahamas and a bit isolated from the quant community, so being able to connect with CQF delegates and the broader quant community was very helpful. Finally, I felt that the range of topics covered in the CQF appealed to me, and the coursework was complementary to the studies I had completed in the CFA program. I also found the online platform incredibly useful. For me, the time zone wasn't a big issue, but I appreciated being able to take a high-quality course exclusively online. Now it's more of a standard option in professional education, but they certainly were breaking new ground then.
Where did the quant finance path lead you after that?
I spent a few more years at the fund in the Bahamas and then I went to grad school. I had been working for nine years at that point and wanted to take a break. I ended up completing two degrees: a master’s in computer science at Harvard and an MBA at Columbia University. It was great to be exposed to all the programs and professors after those years of practical experience. At Harvard, I focused on machine learning, and took classes on operating systems and data structures, which are very necessary to that field. I had learned to code while I was in London and the Bahamas, and this was a good way to strengthen my understanding of the bigger picture in programming.
One thing people often ask on the programming side is that while Python is a leading language for data science, they wonder if learning C or C++ is also a good idea? What's your take on programming languages?
I think it depends on your appetite for programming in general and on your areas of specialization. There's are many benefits from learning C and C++. They will give you a much richer understanding of how programs operate and it's a great background to have. But is it strictly necessary? No. Certainly in most quant funds, a lot of the prototyping is done in Python, and then there are specialists who implement things in C or C++. I've seen that work quite well, so, people shouldn't feel like they must learn C to be successful in this type of career. That said, they will certainly be better off if they take some classes in C and its variants because there is a richness of information that comes from understanding the internals of the systems on which C and C++, for example, are built.
What have you been doing since you finished your academic programs?
After grad school I was lucky enough to join Google as a Data Scientist. The role was originally called “Quantitative Analyst” when I went in, but they changed the title to Data Scientist while I was there. I did that for five years and then about a year and a half ago, I left to join Balyasny Asset Management as the Head of Data Science for a new Growth Equity Fund, which is focused on investing in private companies that are on the path to doing an IPO.
What would you advise people who are getting into quant finance now, from what you've seen in various work environments?
I would say that in quant finance, the world is coming to us, but the definitions keep changing. Alternative data and data analysis are in fashion now and there's never been a greater need for quants working in these areas. Even in the world of fundamental investing, this is something I see on a regular basis. Even at Columbia Business School, one of the traditional homes of the value investing philosophy, they are making Python classes mandatory. Enrolling in high-quality, well-established quant programs like the CQF is more important than ever because everyone is speaking our language these days, but few truly understand it.
Find out more about the CQF program
To discover how the CQF program could help you go further in your career, download a brochure or register to join an information session.